Routable CEO Omri Mor sat down with Christian Wattig, a former finance leader turned instructor, to talk about how AI tools are making the lives of finance leaders easier and helping them drive growth and profitability. Below are the key questions Christian weighed in on, edited for clarity (with a few thoughts from Omri popped in!).
When we discuss AI for finance, there are really two different sub-topics of AI that we need to talk about. There is machine learning and generative AI. Can you explain these two first before we go deeper into it?
Christian: The definition of AI is simply that its purpose is to mimic human cognition. Then, there are several sub-fields of AI. Generative AI is what seemingly every software company on the planet currently includes in their products. It’s the chatbots, the ChatGPTs, Geminis, or Microsoft Copilots of the world.
And then there is machine learning. Finance teams have been using machine learning for over a decade now, but many companies I talk to still don’t know how it can help them.
Let’s start with the definition. Machine learning models recognize patterns from historical data to make predictions. In other words, a machine learning model can forecast any data series you throw at it as long as you provide it with enough high-quality historical data.
That can make creating a complex forecast significantly less time-consuming, especially when analyzing large sets of historical data.
Omri’s two cents: We’re in the process of building out Routable’s first AI offering, which will leverage the power of AI to detect and prevent both invoice fraud and human error. We’ll be able to scan invoices for anomalies, identify potential duplicate invoices, and zoom in on honest mistakes. (See below for an example of how this works in practice.)
You mentioned that machine learning has been around for a long time. Can you share an example of when you used it in your finance leadership roles before?
Christian: When I led an FP&A team at a large consumer goods company a few years ago, I was in charge of maintaining the working capital forecast. It was a beast of a forecast with dozens of sub-forecasts for line items and divisions that ladder up to the full company number. Because it was so complex and time-consuming, we chose it to test a machine learning approach for the first time. We decided to outsource the development of the AI model to a third party based in India.
When we received the first results from the new model and compared them a month later to actuals we were excited. The forecast accuracy was significantly better than what we had before. So, I was sure this project would be a resounding success until – the leadership team wanted an explanation for what was driving the remaining variance between what the machine learning model had predicted and the actual results. And I had no idea how to answer that question because I wasn’t involved in building the model.
So, I contacted the data scientist responsible for the model at the third party. I approached it the same way I would analyze a variance coming from a traditional Excel forecast model: I asked him if he could explain how the model works so I could point to what may be causing the variance. He said, “We use an ensemble of different machine learning algorithms. They compete against each other every month, and the best-performing model is used to do the forecast. Specifically, we used machine learning algorithms like DeepAR from Amazon, Temporal Fusion Transformer from Google, and traditional algorithms like Holt-Winters, and Autoregressive Integrated Moving Average models. In total, the ensemble includes 12 different algorithms that compete against each other. We backtest each of them, and then the one with the highest accuracy is chosen.”
I thought, “I can’t say I understood more than half of what he just said.” I followed up with, “Okay, so why do you think there may still be such a significant variance between actual results and what the model has predicted ?” He answered that he had no idea because he didn’t know how our business worked.
So, the situation we were in was that I didn’t understand how the machine learning model worked, and the data scientist didn’t understand how the business worked. How could we explain a variance here? I brainstormed different solutions to this problem. Should we invite the data scientist to our office so we can explain how the business works? Should we ask someone on our team to take a course on machine learning and data science? Was it even possible to learn this without getting a degree in math or computer science?
It took me a while, but I finally realized I was looking at the problem all wrong. In fact, I was trying to solve the wrong problem entirely. When it comes to machine learning, you don’t explain variances by pointing to flaws in the model itself. The most advanced algorithms are called “black box models” for a reason – even the scientists who built them sometimes don’t understand the conclusions they make.
Actually, I realized it’s all about the inputs. Garbage in, garbage out. To explain variances with machine learning, we need to investigate if the data points we feed the model with are right and sufficient. And the best way to do this is to combine experimentation with an approach called “backtesting”. With AI models, it’s possible to pretend the last year hasn’t happened yet and ask the model to create a forecast. For example, now it’s March of 2025, and I can ask the AI model to forecast 2024 by feeding it with historical data from 2015 to 2023. Then, you can immediately compare how close it was to actual results, adjust the data points, and test it again.
We realized that the forecast accuracy significantly improved when we fed the model with additional data from our inventory systems—data points we had never considered before because doing so would have made our traditional spreadsheet-based models even more complex. Once the leadership team understood this new way of explaining variances, we were off to a great start on the journey of implementing AI and strategic outsourcing.
When someone is considering implementing a machine learning model for the first time, what else do they need to consider?
Christian: When I teach the basics of how machine learning models work, I cover five key concepts. Understanding these won’t turn a finance manager into a data scientist, but it’s enough to be able to collaborate with one.
- Backtesting
Machine Learning models allow us to do what’s almost impossible with traditional modeling: act as if the past hasn’t happened yet. We can run the model using historical data and see how well it would have predicted last year. If today is January 2025, we would feed the model with data for 2023 and ask it to predict 2024. Then, we can compare model outputs with actual results. As a result, we can have multiple models compete with each other and select the one with the lowest variance to reported actuals. - Feature Selection
When the Data Science team presents a model to you, ask which “features” (a term for model inputs) they chose, why they selected them, and which other features they tried. You may have heard the phrase “garbage in, garbage out” before. Computer Scientists use it to remind us that even the best model cannot make valuable predictions if we feed it the wrong inputs. - Overfitting
Overfitting is a modeling error that occurs when a function is too closely aligned to a limited set of data points. As a result, the model is useful in reference only to its initial data set, not to any other data sets. That means a model may have a low forecast accuracy, even if results from backtesting look fine. In that case, experiment with the included features (their nature and number) to reduce the likelihood you are overfitting (e.g., adding unemployment data). - Statistical Significance
When a finding is “statistically significant,” it means you can feel confident that it’s real, not that you just got lucky (or unlucky) in choosing the sample. There are two main factors of statistical significance: the size of the sample and the variation in the underlying population. The larger the sample and the more homogeneous the population is, the more likely it will be that the result of your analysis will have significance. P-value is a metric that measures statistical significance. - Correlation vs Causation
Just because the movements of two variables track each other closely over time doesn’t mean that one causes the other. To differentiate factual causation from mere correlation, we need to consider two things: What’s the causal link or mechanism, and… Is there enough scientific rigor to show a statistically significant correlation?
There are three different options available for implementing a machine learning-based forecast model at your company.
- Build it yourself, which requires an in-house data science team.
- Hire a third-party data science provider who builds and implements a bespoke solution for you.
- Purchase a software subscription with pre-build, “off-the-shelf” machine learning models. Implementing them doesn’t require in-house data science know-how.
Omri’s two cents: Routable was built for AP automation, and recently we’ve been looking for opportunities to help our customers gain efficiencies by building machine learning into our platform. Here are a couple of examples of how this works in an AP context:
- PO Matching: Using machine learning, we can train your invoices to automagically match to your POs.
- OCR Training: Our solution enables customers to train their OCR scanning to increase in accuracy over time. It’s not your mother’s OCR!
Okay, let’s discuss generative AI. How can it help finance teams spot risks and opportunities early?
Christian: Generative AI can help finance teams in two ways: indirectly, by saving them time, and directly, by helping with brainstorming.
When identifying risks and opportunities, finance teams need to dive deep into the numbers, spend time talking to their business partners, and figure out how to connect operational metrics with financials. Otherwise, they just scratch the surface and won’t identify root causes that point to a concrete recommendation for capitalizing on an opportunity or mitigating a risk. But doing that digging takes time. Often, finance teams lack the time to conduct this in-depth analysis. They spend the first one or maybe even two weeks on the month-end close, and then they have to prepare the management reports against tight deadlines and field time-sensitive and ad-hoc analysis or modeling requests. By the time this is done, it can be time to get ready for the next month-end close already.
Generative AI can help finance teams save time in several ways:
- Help with writing emails
- Increase your speed in Excel
- Help make Excel models more robust
- Explain how to get more out of your ERP system and most established tools
- Enable the use of advanced tools like Python and SQL for data management and visualizations
- Now, AI also comes with most software tools in the finance tech stack
However, AI can also help you identify risks and opportunities directly. Let me differentiate between capabilities that already exist today, those currently being actively developed, and those that I expect in the future.
Where we are already fully there:
- Brainstorming partner, for example for:
- Identifying which KPIs to track,
- Which questions to ask business partners,
- Possible reasons for variances
- Possible ways to save costs and become more efficient
- How to answer a tricky question from a business partner
However, keep in mind that if you want to use AI as a brainstorming partner, you must have at least an intermediate understanding of the topic; otherwise, you can easily be misled.
Where we are getting there:
- AI takes on simple analysis tasks. Planning tools build chatbots in their software, which you can ask questions like “Show me the 10 customers that contributed most to the sales miss of last quarter” or “Which P&L line items contributed the most to the cost overrun of last month?”
- Standard variance commentary and corresponding slides with visualizations that get created automatically (however, in a limited way)
I recorded a concrete use case to help you write a successful prompt so AI can help you identify risks and opportunities.
Omri’s two cents: Additional use cases Routable is solving for:
- Detecting invoice fraud: We’re embedding Generative AI into our platform to alert AP teams to potentially fraudulent or incorrect invoices. By catching these issues early, we can help customers prevent payments to bad actors and ensure that they stay compliant.
- Predictive bill coding: Based on previous invoices, Routable learns how to code a bill and makes suggestions in the platform. This frees up valuable time for your finance team and eliminates manual data entry.
Christian: What I expect to be possible in the future:
- AI Agents that you can ask to collect data from various systems, manipulate it, and present it in the way you like. This is technically already possible using data lakes or RPA (robotic process automation). However, these tools tend to be difficult to set up and highly inflexible, so they can’t be used for dynamic ad-hoc analysis.
- Chatbots can analyze large amounts of data across different data sources and apply reasoning to point to signals that are difficult or even impossible to find for humans.
Given all these emerging technologies, how do you see working in finance looking differently in the future than it does today?
Christian: Manual, repetitive tasks will be eliminated completely. That includes reconciliations, data cleaning, data manipulation, report creation, and visualizations. As a result, leaders expect that finance teams deliver more value. And I’m starting to see this already today. Leaders expect finance teams to become strategic partners to the business. That means they are involved in making strategic decisions and are asked to identify changes to trends early enough to be acted upon.
As a result, finance professionals need to be much closer to the business. They need to know how strategies across the company translate to tactics, action plans, and measurement. They need to understand which tactics work and which don’t work and how they become visible in the financials.
So, the demand for strong FP&A and strategic finance professionals will go up rather than down in the future. Because you will always need someone to interpret context and nuances and, in turn, collaborate with other departments to ensure that insights result in the appropriate actions.
I believe that this change will also make finance roles more rewarding. Rather than spending endless hours creating reports that may or may not be used for decision-making, AI helps finance move closer to the business, and as a result, they have a bigger impact in their roles.
Omri’s two cents: Routable’s Hierarchy of Accounting Needs framework (pictured below) perfectly aligns with Christian’s thinking here. Our goal with adding AI to our existing AP automation platform is to significantly reduce the amount of time finance teams are spending on low-value, tedious tasks, freeing up resources for mission-critical, strategic work (i.e., the items at the top of the pyramid).
Thanks to Christian for taking the time to sit down with us for this conversation!
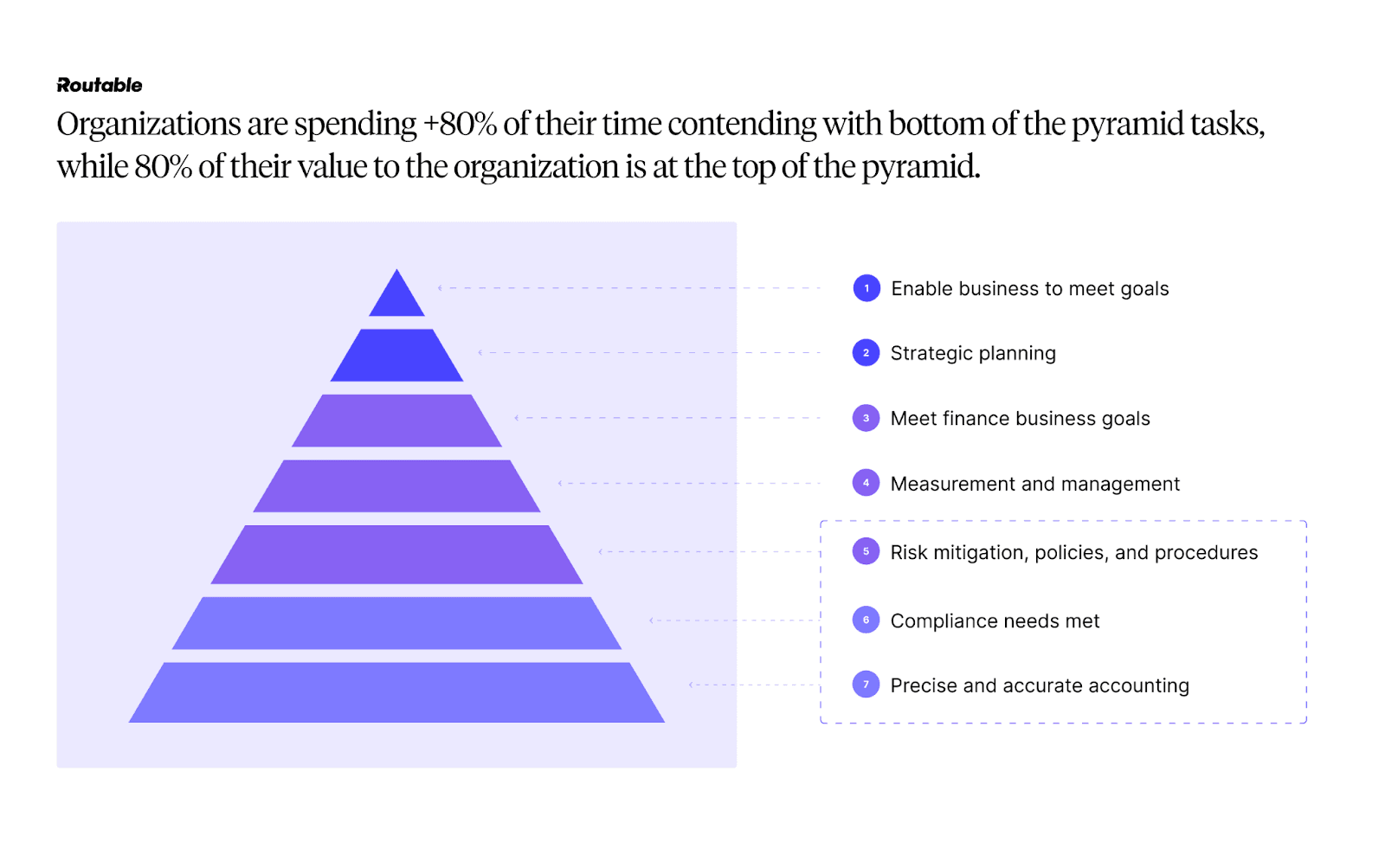